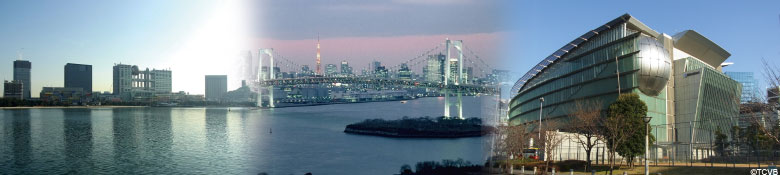
Tutorial Course
Date | May 18, 2015 |
Time | 13:00-17:15 |
Place | Conference Room 1 @ Miraikan |
Domain Adaptation for Visual Recognition
Lecturer: Dr. Vishal M. Patel (University of Maryland, Center for Automation Research)
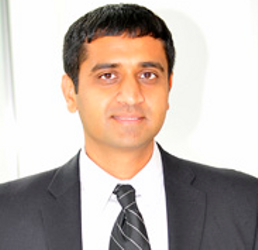
Abstract:
In pattern recognition and computer vision, one is often faced with scenarios where the training data used to learn a model has different distribution from the data on which the model is applied. Regardless of the cause, any distributional change that occurs after learning a classifier can degrade its performance at test time. Domain adaptation tries to mitigate this degradation. In this tutorial, I will give an overview of recent visual domain adaptation methods and their applications in object recognition and biometrics recognition problems.
Schedule: 13:00-15:00
- 15 min: Domain adaptation motivation and background
- 30 min: Feature augmentation-based approaches
- 10 min: Break
- 40 min: Sparse and low-rank models for domain adaptation
- 15 min: Applications in object and biometrics recognition
- 10 min: Q&A
Effective Dataset Construction in Computer Vision
Lecturer: Dr. Kota Yamaguchi (Tohoku University)
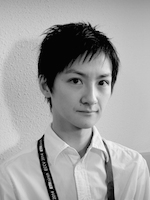
Abstract:
As machine learning methods become the mainstream, we start to see a new challenge in computer vision: lack of data. This tutorial turns our attention to the importance of high-quality data in computer vision. Several approaches have been proposed to efficiently collect and create a high-quality dataset based on human-computation, including a task-specific annotation interface, a human-in-the-loop approach, or a game-based incentive control. Also, crowdsourcing has been utilized not only to assign concrete labels for supervised learning but also to study human behavior in terms of psychological perspective. In this tutorial, I will review various crowdsourcing efforts in computer vision and introduce as a case study the data-driven approach in fashion domain.
Schedule: 15:15-17:15
- 10 min Motivation: importance of data
- 10 min Collecting diverse data
- 40 min Crowdsourcing in vision
- 10 min Break
- 20 min Case study: data-driven human parsing
- 20 min Case study: studying fashion
- 10 min Questions